Synthetic Data Generation Tools: A Revolution in Data Analytics

In today’s data-driven world, the demand for high-quality and diverse data is constantly increasing. However, obtaining such data can be a challenging and expensive task. This is where synthetic data generation tools come into play. These tools use advanced algorithms to create realistic and representative data sets that mimic real-world data. In this blog post, we will explore what synthetic data is, its benefits and challenges, top tools in the market, use cases, future trends, best practices, and real-world examples of using synthetic data.
What is Synthetic Data, and How is it Different?
Synthetic data refers to artificially generated data that imitates real-world data in terms of structure, distribution, and relationships between variables. It is created using mathematical models and algorithms, making it completely different from traditional data collection methods. Unlike real data, synthetic data does not contain any personally identifiable information (PII) or sensitive information, making it safe to use for testing, training, and research purposes.
The main difference between synthetic data and real data lies in their source. Real data is collected from various sources such as surveys, sensors, and social media, while synthetic data is generated by software tools. This makes synthetic data more cost-effective and time-efficient compared to real data. Additionally, synthetic data can be easily manipulated and customized to fit specific use cases, making it a versatile tool for data analysis.
Types of Synthetic Data
There are two main types of synthetic data – fully synthetic and partially synthetic. Fully synthetic data is generated entirely from scratch, without any input from real data. On the other hand, partially synthetic data combines real data with synthetic data to create a hybrid dataset. Partially synthetic data is often used when there is a limited amount of real data available, and the goal is to increase the size and diversity of the dataset.
Top Synthetic Data Generation Tools in the Market
With the increasing demand for synthetic data, there are now numerous tools available in the market. Here are some of the top synthetic data generation tools that you should know about:
Syntho.ai
Syntho.ai is a popular synthetic data generation tool that uses advanced machine learning algorithms to create realistic and diverse datasets. It offers a user-friendly interface and allows users to customize their data based on specific use cases. With its powerful data anonymization capabilities, Syntho.ai ensures that all sensitive information is removed from the generated data, making it safe to use for testing and training purposes.
DataGen
DataGen is a cloud-based synthetic data generation platform that offers a wide range of features such as data customization, data validation, and data visualization. It uses artificial intelligence (AI) and natural language processing (NLP) techniques to generate high-quality data that closely resembles real data. DataGen also provides data privacy and security features, ensuring that all generated data is compliant with data protection regulations.
Hazy
Hazy is an open-source synthetic data generation tool that uses deep learning algorithms to create synthetic data. It offers a simple and intuitive interface, making it easy for users to generate data without any coding knowledge. Hazy also provides data masking and anonymization features, ensuring that all sensitive information is removed from the generated data.
Tonic
Tonic is a powerful synthetic data generation tool that offers a variety of data types, including structured, unstructured, and time-series data. It uses AI and machine learning algorithms to create realistic data that can be used for various purposes such as testing, training, and research. Tonic also offers data privacy and security features, making it a reliable tool for handling sensitive data.
What Synthetic Data Use Cases Should You Know About?
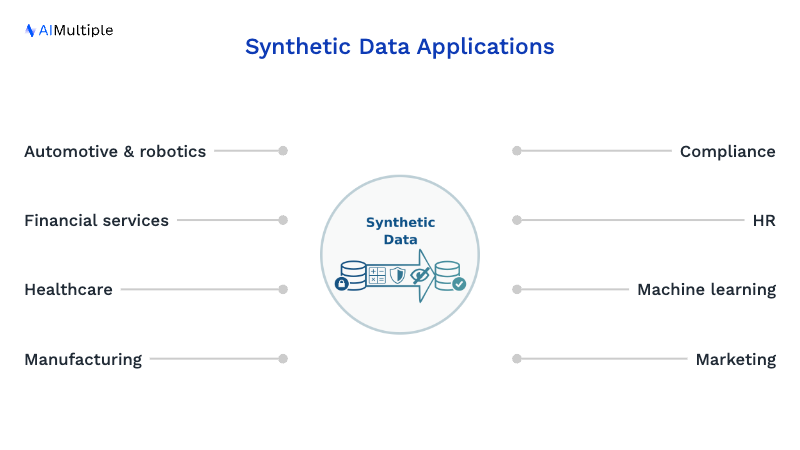
Synthetic data has a wide range of use cases across various industries. Here are some of the most common use cases that you should know about:
Testing and Training Machine Learning Models
One of the most popular use cases of synthetic data is testing and training machine learning models. Synthetic data can be used to create large and diverse datasets, which are essential for training accurate and robust machine learning models. It also allows data scientists to test their models without using real data, reducing the risk of exposing sensitive information.
Data Augmentation
Data augmentation refers to the process of increasing the size and diversity of a dataset by adding new data points. This is particularly useful when there is a limited amount of real data available. Synthetic data can be used to augment real data, creating a larger and more diverse dataset for analysis.
Research and Analysis
Synthetic data can also be used for research and analysis purposes. It allows researchers to manipulate and customize data based on specific scenarios, making it easier to study the impact of different variables on the data. Additionally, synthetic data can be used to fill in missing data points, making it a valuable tool for data analysis.
Benefits of Using Synthetic Data
There are numerous benefits of using synthetic data, some of which include:
Cost-Effective and Time-Efficient
Collecting real data can be a time-consuming and expensive process. With synthetic data, organizations can save both time and money by generating data instead of collecting it from various sources.
Safe to Use
As mentioned earlier, synthetic data does not contain any personally identifiable information or sensitive information, making it safe to use for testing, training, and research purposes. This eliminates the risk of exposing sensitive data and ensures compliance with data protection regulations.
Customizable and Versatile
Synthetic data can be easily manipulated and customized to fit specific use cases. This makes it a versatile tool for data analysis, as it can be used for various purposes such as testing, training, and research.
Challenges of Using Synthetic Data
While synthetic data offers numerous benefits, there are also some challenges that organizations should be aware of before using it. Some of these challenges include:
Lack of Realism
Despite advancements in technology, synthetic data may not always accurately mimic real-world data. This can lead to biased or inaccurate results when using synthetic data for analysis.
Limited Domain Knowledge
Synthetic data is generated by algorithms, which means that it does not have the same level of domain knowledge as humans. This can result in data that does not reflect the complexity and nuances of real data.
Data Quality Issues
The quality of synthetic data depends on the quality of the algorithms used to generate it. If the algorithms are not properly designed or trained, the quality of the generated data may be compromised.
Future Trends in Synthetic Data
As the demand for high-quality and diverse data continues to grow, the use of synthetic data is expected to increase in the future. Here are some of the key trends that we can expect to see in the field of synthetic data generation:
Advancements in AI and Machine Learning
With the rapid advancements in AI and machine learning, we can expect to see more sophisticated algorithms being developed for generating synthetic data. This will lead to more realistic and accurate synthetic data, making it a more reliable tool for data analysis.
Increased Adoption in Healthcare and Finance Industries
The healthcare and finance industries deal with sensitive data, making it challenging to share and use real data for analysis. As a result, we can expect to see an increased adoption of synthetic data in these industries, where data privacy and security are of utmost importance.
Integration with Big Data and Cloud Technologies
As big data and cloud technologies continue to evolve, we can expect to see more integration with synthetic data generation tools. This will make it easier for organizations to generate and analyze large and complex datasets.
Checklist of Considerations When Assessing Synthetic Data Tools
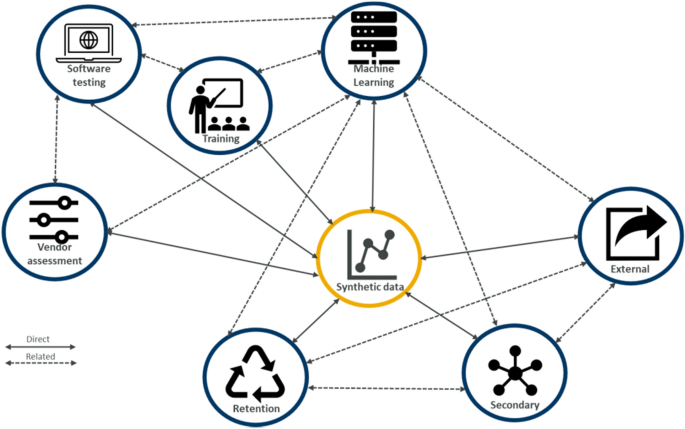
When evaluating different synthetic data generation tools, here are some key considerations that you should keep in mind:
- Data Quality: The quality of the generated data is crucial for accurate analysis. Make sure to assess the algorithms used by the tool and their ability to produce high-quality data.
- Customization Options: Different use cases may require different types of data. Look for tools that offer a wide range of customization options to fit your specific needs.
- Data Privacy and Security: It is important to ensure that the tool has robust data privacy and security features in place to protect sensitive information.
- Integration Capabilities: Consider how easily the tool can be integrated with your existing systems and technologies.
- User-Friendliness: Look for tools that have a user-friendly interface and do not require extensive coding knowledge to generate data.
Best Practices for Synthetic Data Generation
To ensure the best results when using synthetic data, here are some best practices that you should follow:
Understand Your Data Needs
Before generating synthetic data, it is essential to understand your data needs and the specific use case for which the data will be used. This will help you determine the type of data and level of customization required.
Use Multiple Tools
Different tools may excel in different areas, so it is recommended to use multiple tools to generate synthetic data. This will allow you to compare the quality and diversity of the data generated by each tool and choose the best one for your needs.
Validate the Generated Data
It is important to validate the generated data to ensure its accuracy and reliability. This can be done by comparing it with real data or using statistical methods to assess its quality.
Real-World Examples of Using Synthetic Data
Synthetic data has been used in various real-world scenarios, some of which include:
Training Autonomous Vehicles
Autonomous vehicles require large and diverse datasets for training their AI systems. However, collecting such data can be challenging and time-consuming. To overcome this, companies like Waymo have used synthetic data to train their autonomous vehicles, reducing the time and cost involved in data collection.
Improving Healthcare Analytics
Healthcare organizations often face challenges when it comes to sharing and analyzing patient data due to privacy concerns. To address this issue, researchers at MIT have developed a synthetic data generation tool that creates realistic medical records without any sensitive information, making it easier to share and analyze data for research purposes.
Enhancing Fraud Detection Systems
Financial institutions use fraud detection systems to identify and prevent fraudulent activities. However, these systems require large and diverse datasets to accurately detect fraud. To overcome this, companies like Capital One have used synthetic data to augment their real data, creating a larger and more diverse dataset for their fraud detection systems.
Conclusion: Synthetic Data – A Revolution in Data Analytics
In conclusion, synthetic data is a powerful tool that offers numerous benefits for data analysis. It is cost-effective, safe to use, and customizable, making it a versatile tool for various use cases. While there are some challenges associated with using synthetic data, advancements in technology and algorithms are expected to address these issues in the future. With the increasing demand for high-quality and diverse data, we can expect to see a continued growth in the adoption of synthetic data in various industries. As such, it is important for organizations to stay updated on the latest trends and best practices in synthetic data generation to leverage its full potential for data analytics.
Also visit for more blogs at : 44th Street Restaurants and Dining Guide